Recent advances in Artificial Intelligence (AI) have enabled exciting applications, which are now playing important roles in everyday life ranging from language translation and image processing to recommender systems and autonomous driving. Most applications are based on Machine Learning (ML), which achieved great successes due to increasingly available computational resources and data. The current trend of AI offers numerous opportunities to contribute within areas of research, theory, technology, and application. In TRAIL, we investigate different directions of AI and ML to provide novel methods and insights to pave the way for future applications and technologies.
Structure
1. Overview
In TRAIL, we regard the term intelligence with respect to the behaviour of an entity. For simplification we assume an entity to be intelligent if it is able to learn from past experience, to think about future events or actions, and to act according to its knowledge, thoughts, and interaction with other entities. Our research in TRAIL focuses on these three main aspects:
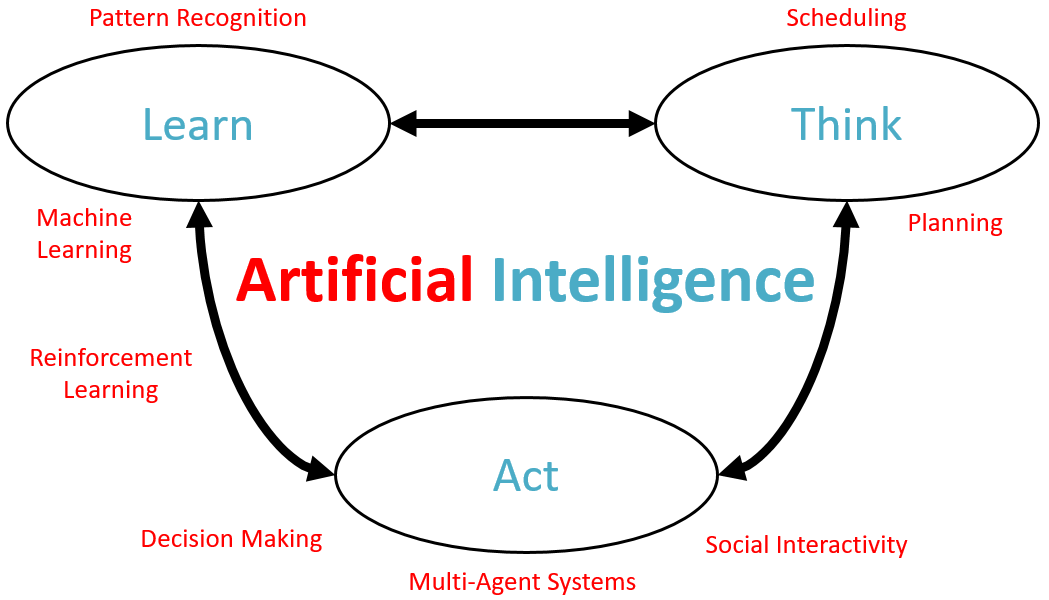
Learn
Learning is the process of extracting knowledge from data which represents past experience. The knowledge can be used to identify salient patterns or structures in data or to make predictions. Machine Learning is currently the most active field in AI and has achieved tremendous progress in various domains over the last decade.
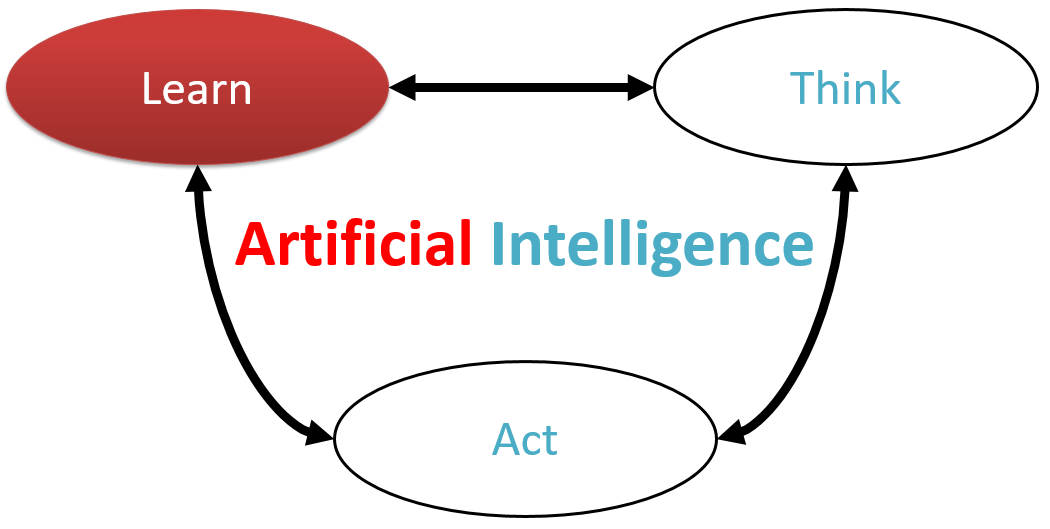
Selected Publications
- Robert Müller, Steffen Illium, Thomy Phan, Tom Haider, and Claudia Linnhoff-Popien, „Towards Anomaly Detection in Reinforcement Learning“, in AAMAS 2022 Blue Sky Ideas.
Think
The goal of Thinking is to solve problems via explicit reasoning given a problem model, rules, or a simulator. Planning and Scheduling represent common classes of problem solvers and are often used for complex tasks like routing, task allocation, and decision making.
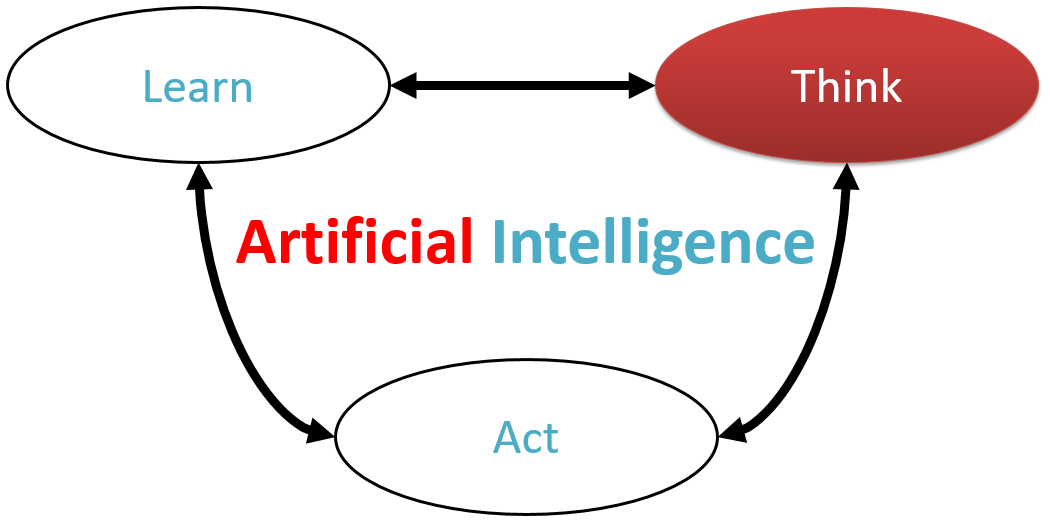
Selected Publications
- Thomy Phan, Thomas Gabor, Robert Müller, Christoph Roch, and Claudia Linnhoff-Popien, „Adaptive Thompson Sampling Stacks for Memory Bounded Open-Loop Planning“, in IJCAI 2019, pp. 5607-5613
- Thomas Gabor, Jan Peter, Thomy Phan, Christian Meyer, and Claudia Linnhoff-Popien, „Subgoal-Based Temporal Abstraction in Monte-Carlo Tree Search“, in IJCAI 2019, pp. 5562-5568
- Thomy Phan, Lenz Belzner, Marie Kiermeier, Markus Friedrich, Kyrill Schmid, and Claudia Linnhoff-Popien, „Memory Bounded Open-Loop Planning in Large POMDPs using Thompson Sampling“, in AAAI 2019, pp. 7941-7948
Act
Acting of AI systems involves the process of making intelligent decisions based on knowledge learned from prior experience or explicit reasoning. Acting is also influenced by coexisting AI systems e.g., in a multi-agent system. Social interaction with humans is important to integrate AI into our everyday life.
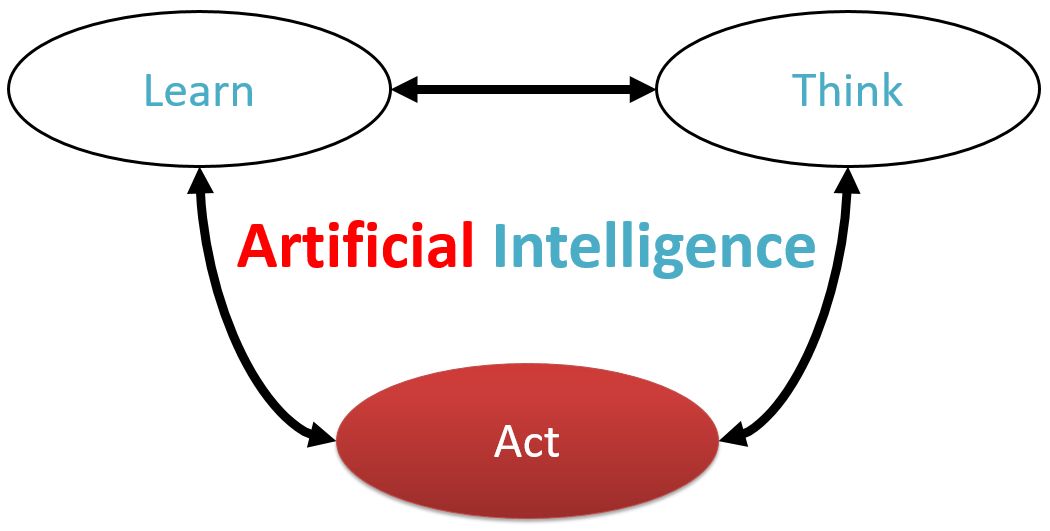
Selected Publications
- Philipp Altmann, Leonard Feuchtinger, Fabian Ritz, Jonas Nüßlein, Claudia Linnhof-Popien, Thomy Phan, „CROP: Towards Distributional-Shift Robust Reinforcement Learning using Compact Reshaped Observation Processing“, in 32nd International Joint Conference on Artificial Intelligence (IJCAI ’23), 2023.
- Thomy Phan, Fabian Ritz, Philipp Altmann, Maximilian Zorn, Jonas Nüßlein, Michael Kölle, Thomas Gabor, and Claudia Linnhoff-Popien, „Attention-Based Recurrence for Multi-Agent Reinforcement Learning under Stochastic Partial Observability“ in Proceedings of the 40th International Conference on Machine Learning (ICML), 2023.
- Thomy Phan, Felix Sommer, Philipp Altmann, Fabian Ritz, Lenz Belzner, and Claudia Linnhoff-Popien, „Emergent Cooperation from Mutual Acknowledgment Exchange“, in AAMAS 2022.
- Thomy Phan, Fabian Ritz, Lenz Belzner, Philipp Altmann, Thomas Gabor, and Claudia Linnhoff-Popien, „VAST: Value Function Factorization with Variable Agent Sub-Teams“, in NeurIPS 2021.
- Kyrill Schmid, Robert Müller, Lenz Belzner, Johannes Tochtermann, and Claudia Linnhoff-Popien, „Stochastic Market Games“, in IJCAI 2021, pp. 384-390
- Thomy Phan, Lenz Belzner, Thomas Gabor, Andreas Sedlmeier, Fabian Ritz, and Claudia Linnhoff-Popien, „Resilient Multi-Agent Reinforcement Learning with Adversarial Value Decomposition“, in AAAI 2021, pp. 11308–11316
- Thomy Phan, Thomas Gabor, Andreas Sedlmeier, Fabian Ritz, Bernhard Kempter, Cornel Klein, Horst Sauer, Reiner Schmid, Jan Wieghardt, Marc Zeller, and Claudia Linnhoff-Popien, „Learning and Testing Resilience in Cooperative Multi-Agent Systems“, in AAMAS 2020, pp. 1055-1063
- Thomy Phan, Kyrill Schmid, Lenz Belzner, Thomas Gabor, Sebastian Feld, and Claudia Linnhoff-Popien, „Distributed Policy Iteration for Scalable Approximation of Cooperative Multi-Agent Policies (Extended Abstract)“, in AAMAS 2019, pp. 2162-2164
- Thomy Phan, Lenz Belzner, Thomas Gabor, and Kyrill Schmid, „Leveraging Statistical Multi-Agent Online Planning with Emergent Value Function Approximation“, in AAMAS 2018, pp. 730-738
See all publications here.
2. Projects and Activities
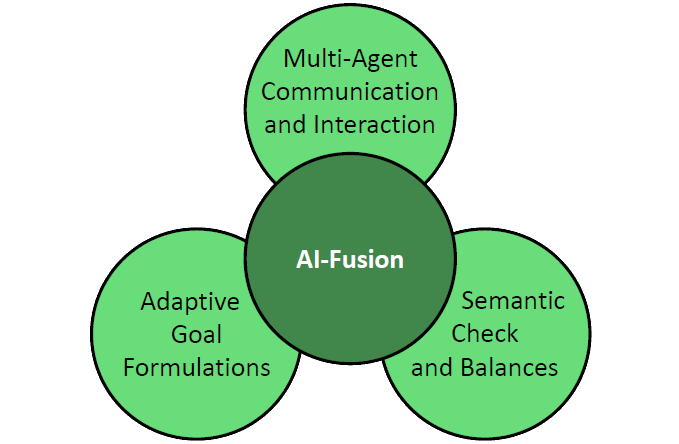
AI-Fusion (Fraunhofer IKS)
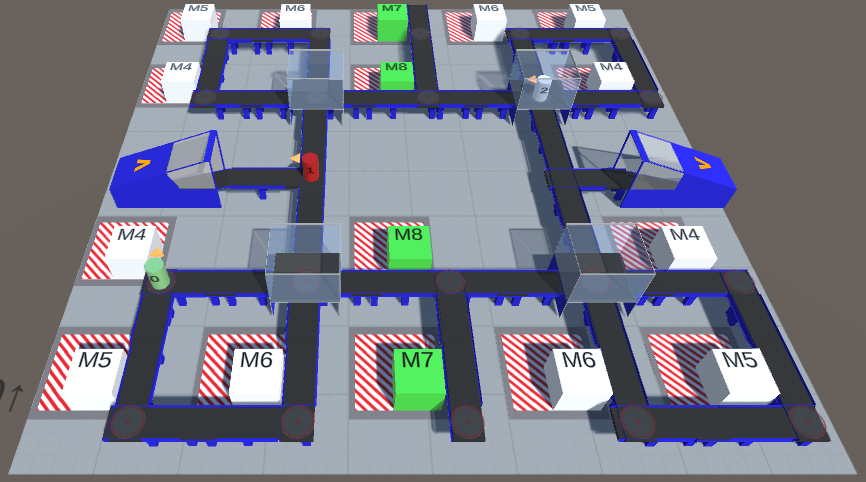
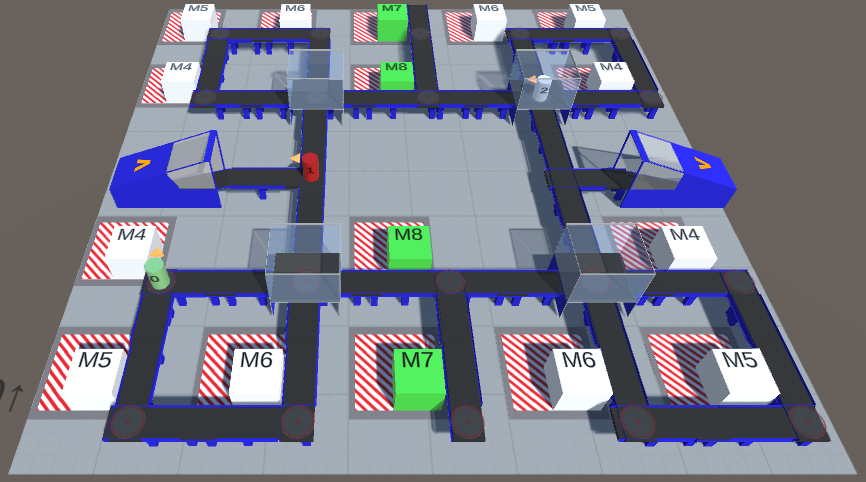
Learning 2 (Siemens)
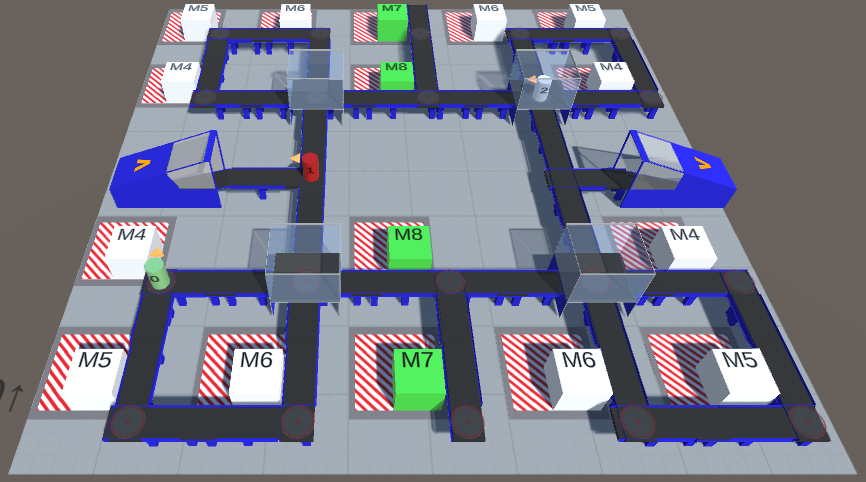
Learning 2 (Siemens)
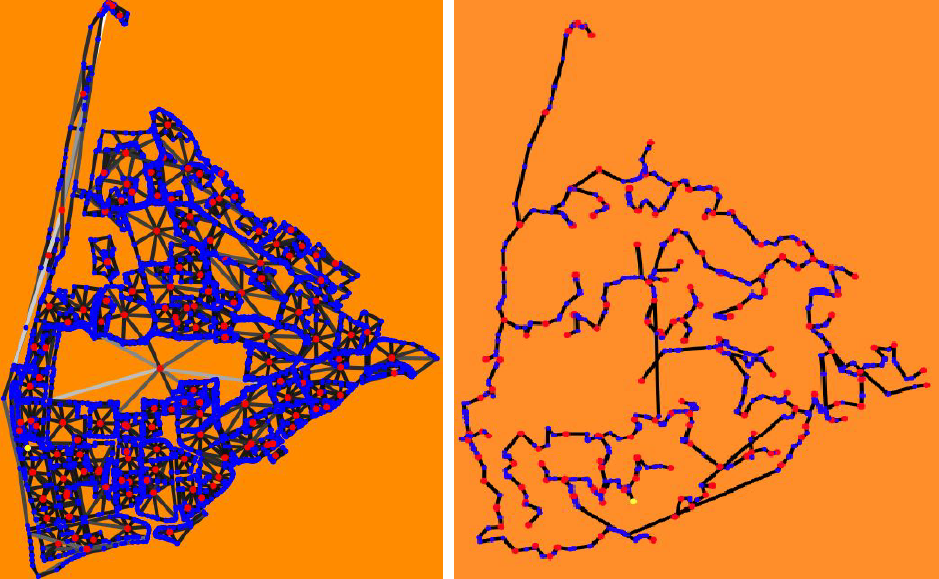
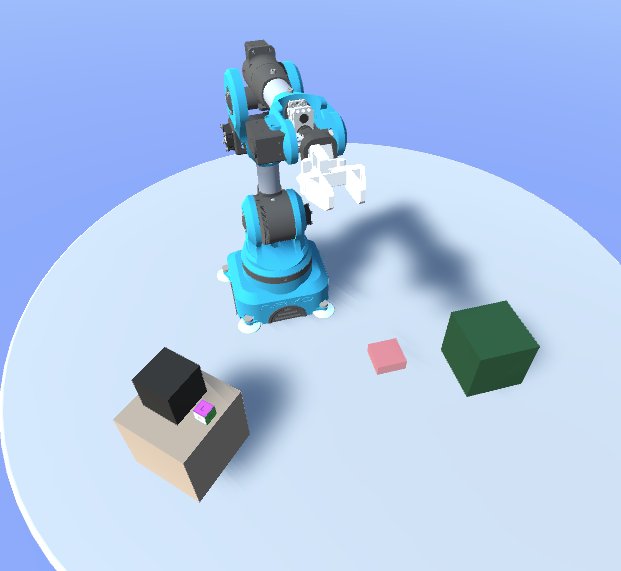
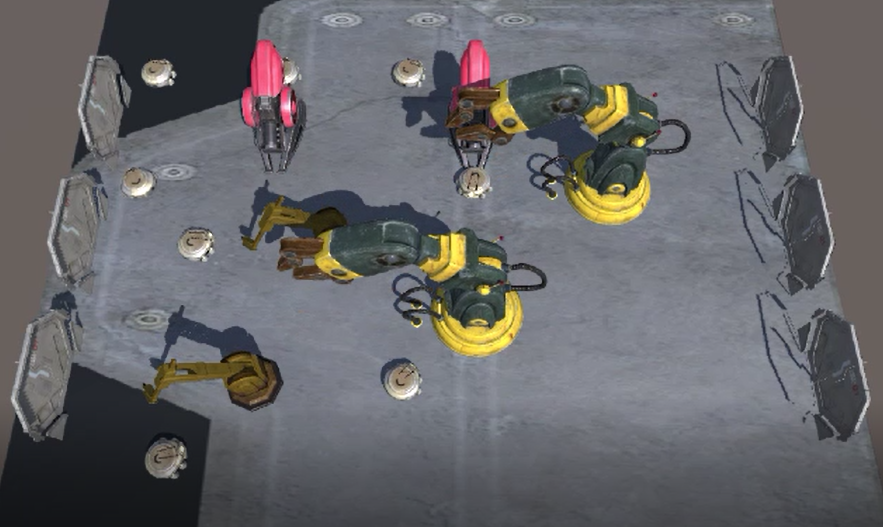
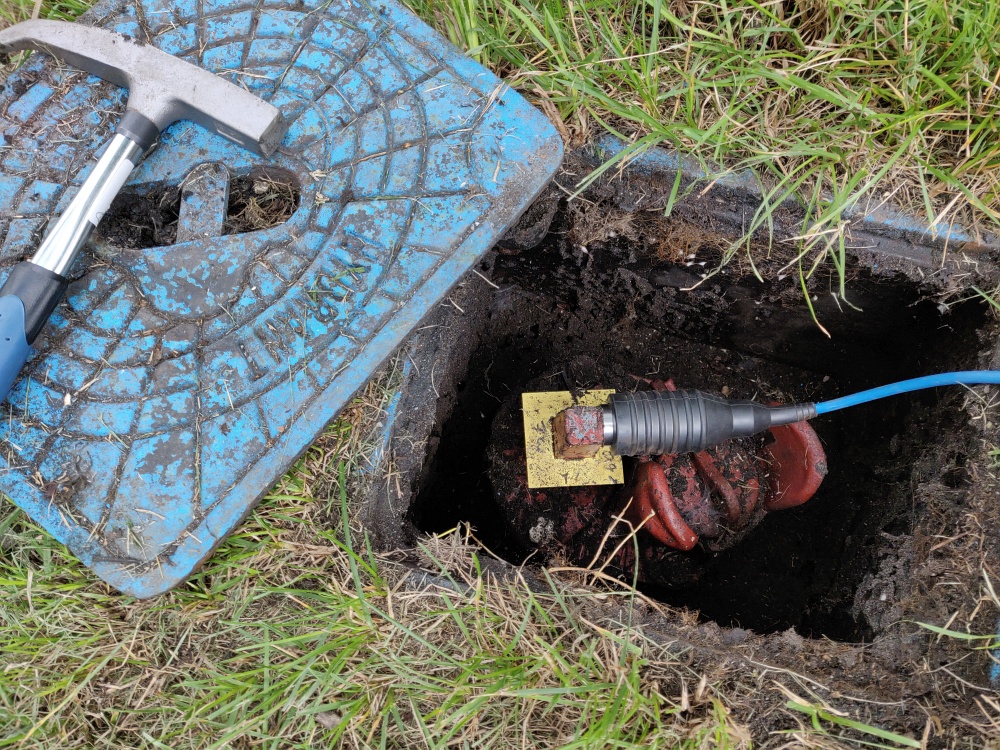
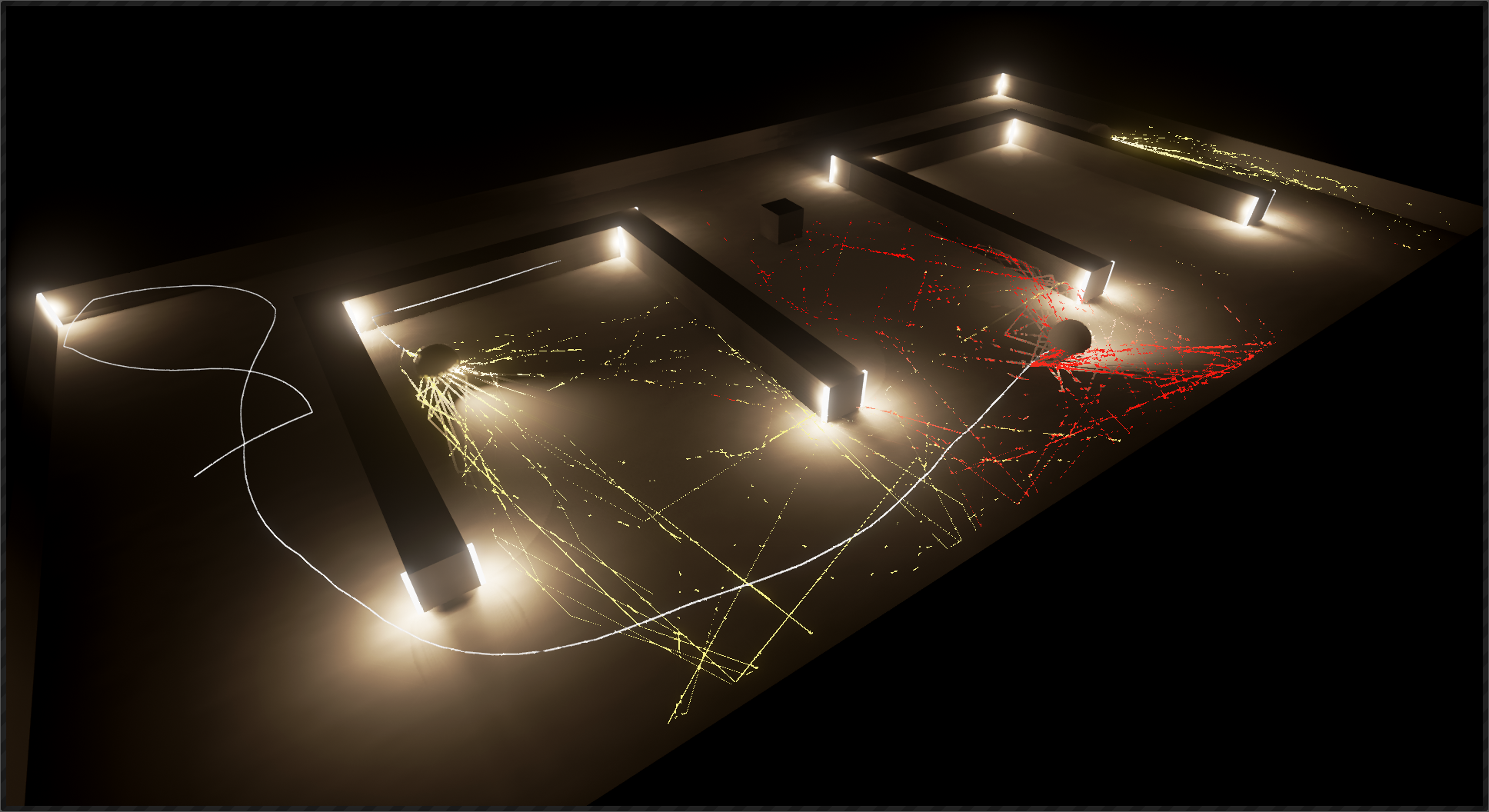
3. Teaching
We offer a diverse set of lectures and practical courses with each covering different areas of AI. Students eligible to attend these courses have the opportunity to learn about the theoretic aspects of AI and to practice their skills by implementing and evaluating algorithms. Courses are occasionally held in cooperation with industrial partners to enable our students to gain further experience. Especially skilled students are offered to actively participate in TRAIL by carrying out individual research projects or writing their theses about exciting topics in the field of AI.
- Intelligent Systems (Lecture): SS 2019, SS 2020, WS 2022/23, WS 2023/24
- Natural Computing (Lecture): SS 2022, SS 2023, SS 2024
- Computational Intelligence (Lecture): WS 2022/23, WS 2024/24
- Autonomous Systems (Practical Course): SS 2019, WS 2019/20, SS 2020, WS 2020/21, SS 2021, WS 2021/22, SS 2022, WS 2022/23, SS 2023, WS 2023/24, SS 2024
- Affective Computing (Practical Course): SS 2017, WS 2017/18, SS 2018, WS 2018/19, SS 2019, WS 2019/20, SS 2020, WS 2020/21, SS 2021, WS 2021/22, SS 2022, WS 2022/23, SS 2023, WS 2023/24, SS 2024
- Innovative Mobile Applications (Practical Course): WS 2015/16, SS 2016, WS 2016/17, SS 2017, WS 2017/18, SS 2018, SS 2019
- Artificial Intelligence (Seminar): SS 2017
- Artificial Intelligence (Working Group): SS 2019, WS 2019/20, SS 2020, WS 2020/21, SS 2021, WS 2021/22, SS 2022, WS 2022/23, SS 2023, WS 2023/24, SS 2024